Remy - The smart assistant for commercial kitchens
Premise
It had been my father’s dream to open a restaurant. At 56, emboldened by passion and spirit of entrepreneurship, he opened an Indian restaurant in Dallas.
During my MBA break, I spent two months working there, hoping to help streamline operations. I soon discovered a major pain point - our reliance on a time-consuming, manual process to onboard and train new chefs. It severely limited our capacity to expand. I set out to solve this problem with technology - envisioning an ML-based solution that could replicate our head chef's knowledge.
Problem
Indian cuisine relies heavily on complex cooking techniques and long sequences of steps. Mastery takes years under a skilled chef. As a new restaurant, quickly training novice cooks was imperative, but extremely difficult. Our head chef could only directly train one or two at a time.
We desperately needed to shorten onboarding from months to weeks for faster expansion. What we needed was a scalable system to train cooks. One that deeply ingrained the nuance and rhythm of our recipes. I envisioned a solution that augmented human training ability, not replacing it.
Current Solutions in the Market
I explored what solutions already existed in the market aiming to solve similar training and onboarding needs. Many products provided digital repositories for recipes and basic kitchen timer functions. However, these were still fully reliant on the innate skill of the individual cook to produce quality outcomes. More advanced options, like fully robotic meal preparation, were prohibitively expensive for most restaurants to implement. The technology is not yet adaptable and affordable enough to truly replicate human-level cooking techniques and mastery.
It was clear there was ample space for innovation of a system that strikes a balance between automation and human effort, leveraging both at their respective strengths.
Product
I conceived an augmented kitchen assistant "Remy" that uses thermal sensors, computer vision and Machine Learning to guide novice cooks in preparing dishes.
- How it works:
- Restaurant owner uploads recipes
- Remy extracts cook times, temperatures, and weights for each step
- Each step gets converted into on-screen instruction via the projector
- Sensors track the chef's progress
- Thermal for monitoring stove & meat temperature
- Weight sensors to monitor ingredient quantities
- Cameras visually confirm each step
- ML model learns from the chef mistakes
- The model improves over time as data aggregates
- Export tuned models to expand to multiple restaurants with similar cuisine
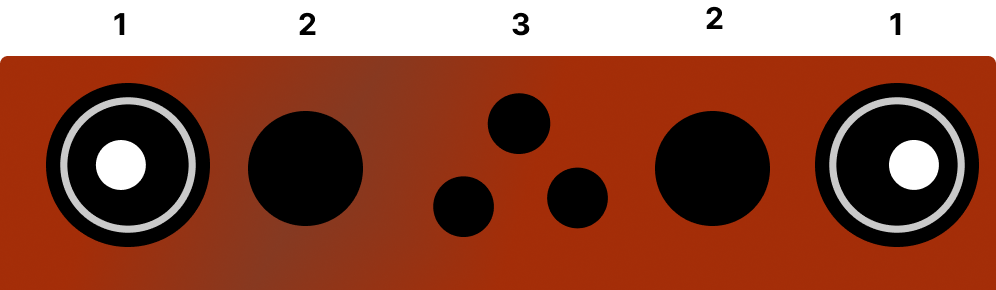
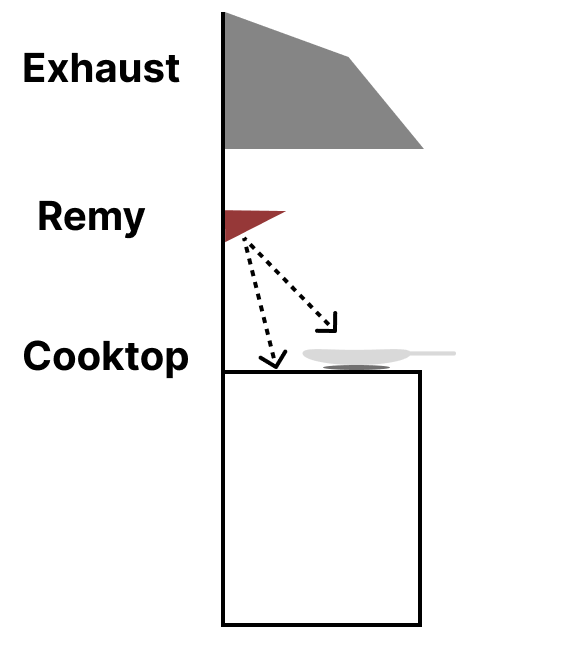
Challenges
- Upon testing, several flaws emerged:
- Space constraints: Most kitchens lacked counter space for projections and sensors.
- Heat/Condensation: Kitchen conditions interfered with sensors and lenses. Frequent cleaning was required which interrupted kitchen operations.
- Costs: Restaurants struggling during COVID were wary of any investments.
- Chef resistance: Some saw it as their replacement and sabotaged the device.
These were just some of the flaws that emerged among many other smaller nuances.
Conclusion
This idea failed to translate successfully from concept to reality. Deployment would be enormously complex even if engineering obstacles were solved. And pushback from chefs posed operational challenges.
I remain compelled by the problem but this proposed solution was not feasible. The journey continues to find alternative approaches.
hi@chaikulkarni.com